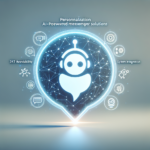
How AI-Powered Messenger Solutions Enhance Sales Interactions
January 27, 2025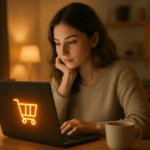
The Role of Machine Learning in Personalizing Customer Experiences During Online Shopping
April 16, 2025Generative Artificial Intelligence (AI) highlights the uses of generative AI by advancing from a research novelty to a transformative force impacting creativity and innovation. Consequently, it produces novel, imaginative outputs—from realistic images to meaningful text—unlocking new possibilities. This article highlights the uses of generative AI and explores its essential principles, significant innovations, and how it reshapes various industries.
Understanding Generative AI
Generative AI is a machine learning model used to create new content, such as images, audio, text, or even 3D models. This content is extremely similar to existing data. As a result, the diverse uses of generative models across various applications demonstrate their adaptability. The most common forms of generative models are:
Types of Generative Models
- Generative Adversarial Networks (GANs)
GANs, introduced by Ian Goodfellow and others in 2014, consist of two neural networks—a generator and a discriminator—that work together. The generator creates new samples, and the discriminator evaluates them against real data. Over time, the generator can produce highly realistic synthetic outputs (Goodfellow et al., 2014). - Variational Autoencoders (VAEs)
VAEs learn low-dimensional data representations, also known as “latent space”. They generate new samples by decoding points from this space. This process enables interpolation between data points, thereby controlling some features of the generated material (Kingma & Welling, 2013). - Transformers for Text Generation
GPT (Generative Pre-trained Transformer) and other transformer models succeed in generating fluently readable, human-like text. They employ attention mechanisms to understand and generate human language (Vaswani et al., 2017; Brown et al., 2020).
Key Innovations and Milestones
- Artistic Creation and Image Generation
The introduction of GANs highlights the potential of AI-generated artwork. As a result, artists now collaborate with AI to produce unique creations, from paintings to digital art. Websites like Stable Diffusion (Ramesh et al., 2021) and DALL·E allow people to create images from detailed text descriptions. This accessibility enables everyone to visualize ideas easily. - Text Generation and Virtual Assistants
Large language models (LLMs), such as OpenAI’s GPT series, revolutionize natural language processing. They produce coherent, context-aware text. Therefore, these models power chatbots, virtual assistants, content-generation tools, and translation systems. As a result, they become a valuable resource for writers, journalists, educators, and businesses seeking fast, high-quality drafts or insights. - Music and Audio Synthesis
Generative AI also reshapes music composition and audio design. Tools like OpenAI’s Jukebox use deep networks to generate unique songs or styles reminiscent of specific artists. Consequently, commercial music creators use generative models to craft new soundscapes, jingles, and background music for films and ads. - Healthcare and Drug Discovery
Outside the arts, generative models are recognized in scientific research. They master complex patterns in molecular and biological data, producing novel drug candidates or designing proteins with specific attributes. This streamlines the drug-discovery process, potentially saving time and cost. - 3D Design and Virtual Worlds
Generative AI advances into 3D modeling, helping game creators and architects develop realistic environments quickly. By training on large 3D data, models can generate objects, characters, and virtual worlds, creating new possibilities for film, games, and virtual reality.
Transforming Creativity and Innovation
- Empowering Non-Experts
Generative AI democratizes creativity by lowering barriers to entry in the creative industry. Morover, individuals without artistic training can generate original art, music, and written content within minutes. Thus, this accessibility encourages experimentation and accelerates innovation. - Enhancing Human-AI Collaboration
Instead of replacing human imagination, generative AI acts as a partner. AI systems inspire humans by offering a stream of new ideas or prototypes. Consequently, this collaboration results in a creative process where humans are curators, editors, or final decision-makers and AI serves as a powerful assistant. - Igniting New Business Models
Companies embed generative AI in their products and offerings, from marketing content platforms to customizable design software. Therefore, new businesses in the generative AI space demonstrate vast business potential. This suggests that generative AI will continue to create new markets and reshape consumer experiences. - Driving Research and Development
In research, generative models provide a sandbox for new machine learning approaches. They trigger studies into adversarial training, privacy-preserving data generation, and robust architectures. Improvements might lead us toward multi-modal systems, robotics, and artificial general intelligence (AGI).
Ethical Problems and Technical Obstacles
The promise of generative AI is huge but comes with ethical and technical challenges:
- Data Privacy and Bias
AI content quality depends on training data, which can be biased or personal, leading to biased or offensive outputs. - Misinformation and Deepfakes
Generative AI can create realistic images, videos, or text, making disinformation easier. Detecting AI-generated forgeries is now crucial. - Intellectual Property Rights
Training AI models on copyrighted material raises questions about content ownership. - Environmental Impact
Large model training consumes significant computational power, impacting the technology’s carbon footprint.
Collaborative efforts by policymakers, researchers, and industry leaders are essential to ensure responsible development and use of generative AI.
Conclusion
Generative AI is breaking new ground for creativity. It empowers everyone to produce high-quality original content and creates breakthroughs in fields like pharmaceutical development. As a co-creative partner, it’s opening an era where human imagination and machine intelligence combine to tackle difficult challenges and enrich our cultural landscape. As we explore the potential of generative AI, it’s vital to prioritize ethics, transparency, and responsible development to enable future creativity and innovation.
References
- Brown, T., Mann, B., Ryder, N., Subbiah, M., Kaplan, J., Dhariwal, P., … & Amodei, D. (2020). Language Models are Few-Shot Learners. arXiv preprint arXiv:2005.14165.
- Goodfellow, I., Pouget-Abadie, J., Mirza, M., Xu, B., Warde-Farley, D., Ozair, S., … & Bengio, Y. (2014). Generative Adversarial Networks. arXiv preprint arXiv:1406.2661.
- Kingma, D. P., & Welling, M. (2013). Auto-Encoding Variational Bayes. arXiv preprint arXiv:1312.6114.
- Ramesh, A., Pavlov, M., Goh, G., Gray, S., Voss, C., Radford, A., … & Sutskever, I. (2021). Zero-Shot Text-to-Image Generation. arXiv preprint arXiv:2102.12092.
- Vaswani, A., Shazeer, N., Parmar, N., Uszkoreit, J., Jones, L., Gomez, A. N., … & Polosukhin, I. (2017). Attention Is All You Need. arXiv preprint arXiv:1706.03762.